How machine learning is transforming educational assessments
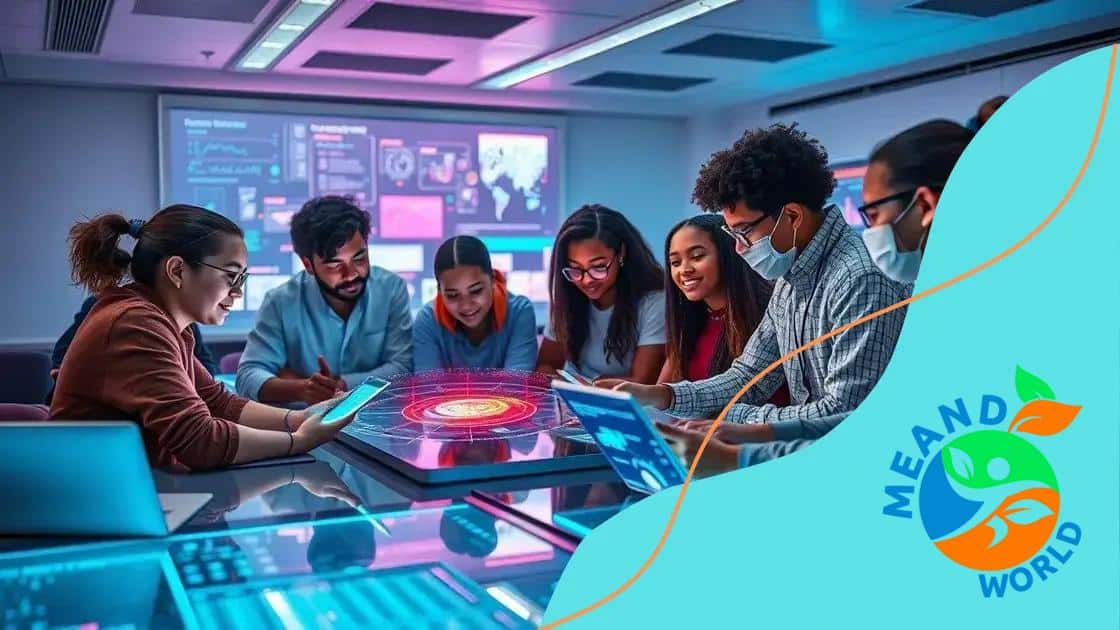
Machine learning is transforming educational assessments by enabling personalized learning experiences, providing continuous feedback, and utilizing data-driven insights to enhance student engagement and outcomes.
How machine learning is transforming educational assessments is reshaping the way students learn and teachers evaluate performance. We’ve all felt the pressure of traditional testing methods, right? Imagine a world where assessments adapt to each student’s needs.
Understanding machine learning in education
Understanding machine learning in education is essential for grasping how technology can improve learning processes. Educators are now using algorithms that learn from data to enhance teaching and boost student success.
What is Machine Learning?
Machine learning is a branch of artificial intelligence that teaches computers to learn from experience. In an educational context, this means algorithms analyze student performance and adapt instruction accordingly.
How It Works
The process begins with gathering data from students’ learning experiences. This data can include test scores, participation, and even online activity. The algorithms then identify patterns in this data to help predict future performance.
- Data collection from various sources
- Pattern recognition through algorithms
- Predictive analysis for personalized learning
Once the data is analyzed, teachers receive insights that allow them to tailor their methods. For example, if a student struggles with math concepts, the system can recommend specific resources or interventions.
Benefits of Machine Learning in Schools
Implementing machine learning provides numerous advantages. It facilitates personalized learning experiences, ensuring each student receives the support they need. This technology can also highlight at-risk students who may need additional help.
- Personalized learning for all students
- Early intervention for at-risk learners
- Efficient lesson planning
- Enhanced assessments based on individual performance
In summary, gaining a clear understanding of how machine learning operates in education opens doors to innovative strategies. As we dive deeper into this topic, it becomes increasingly clear that these advancements have the potential to reshape classrooms.
Benefits of adopting machine learning
The benefits of adopting machine learning in education are profound. Schools that leverage this technology can transform their teaching methods and improve student outcomes significantly.
Personalized Learning
One of the most significant advantages is the ability to deliver personalized learning experiences. By analyzing a student’s progress, machine learning systems can tailor educational content to fit their specific needs. This means that each student can learn at their own pace.
Enhanced Engagement
Using machine learning increases student engagement. Customized approaches keep students interested in their own learning journeys. As students receive feedback tailored to their learning style, they often find the material more relatable and enjoyable.
- Dynamic feedback: Helps students understand their strengths and areas for improvement.
- Interactive resources: Creates a more immersive learning environment.
- Motivational tools: Encourages self-directed learning and growth.
Moreover, machine learning can support teachers by providing detailed insights into classroom dynamics. With the right tools, educators can easily identify trends in student performance. For instance, they may notice a group of students struggling with a particular topic, allowing them to address it promptly.
Efficiency in Administrative Tasks
Adopting machine learning can also streamline administrative tasks. Automated grading and reporting can save educators a significant amount of time, enabling them to focus more on teaching rather than paperwork.
- Automated assessments: Reduces grading time.
- Data analysis: Provides valuable insights for decision-making.
- Resource allocation: Helps in distributing resources effectively based on need.
With these benefits, it’s clear that machine learning can create a more efficient educational system. Schools that adopt this technology can look forward to a bright future in enhancing the learning experience.
Examples of machine learning applications
Examples of machine learning applications in education showcase its potential to revolutionize learning. Many schools are now leveraging technology to enhance student engagement and improve results.
Adaptive Learning Platforms
One popular example is adaptive learning platforms. These tools adjust to each student’s learning pace. For instance, if a student struggles with a specific math topic, the platform can provide additional resources or practice problems tailored to that area.
Intelligent Tutoring Systems
Machine learning powers intelligent tutoring systems that offer personalized assistance. These systems analyze student interactions and learn their preferences, ensuring that each learner receives the right help at the right time.
- Customized feedback: Provides immediate responses to students.
- Learning paths: Guides students through personalized educational journeys.
- 24/7 availability: Offers support whenever needed.
Another example includes automated grading systems. These platforms use machine learning algorithms to evaluate assignments and tests. They save educators time while ensuring more consistent grading standards.
Data-Driven Insights
Furthermore, by analyzing data from assessments, schools can gain valuable insights into student performance. This analysis helps identify which teaching methods resonate most effectively with different student groups.
- Performance tracking: Monitors student progress in real-time.
- Engagement metrics: Evaluates how actively students participate.
- Curriculum adjustments: Informs necessary changes based on performance trends.
Incorporating these machine learning applications elevates the educational experience, making learning more engaging and effective than ever before.
Challenges in implementing machine learning
Challenges in implementing machine learning in education can be significant. While the technology offers many benefits, several hurdles may slow down its adoption.
Data Privacy Concerns
One of the primary challenges is data privacy. Schools must protect students’ personal information while using machine learning. Parents and educators worry about how data is collected and stored.
Integration with Existing Systems
Another issue is the integration of new technologies with existing educational systems. Many schools use outdated software that may not be compatible with advanced machine learning tools. This can lead to frustration and inefficiencies.
- Compatibility: Ensuring new tools work with old systems is crucial.
- Training: Teachers must learn to use new technologies effectively.
- Resource allocation: Schools need adequate funding to upgrade systems.
Additionally, there is the challenge of training educators. Many teachers may not be familiar with machine learning and need ongoing support to feel confident using these tools in the classroom.
Cultural Resistance
Cultural resistance can also hinder implementation. Some educators and school leaders may be skeptical about technology’s effectiveness. They might prefer traditional teaching methods over adopting new ones.
- Mindset shift: Changing long-held beliefs about education takes time.
- Engagement: Involving all stakeholders is essential for acceptance.
- Awareness: Raising awareness about the benefits of machine learning helps.
Addressing these challenges requires a collaborative effort from teachers, administrators, and tech providers. With the right approach, schools can overcome these barriers and harness the full potential of machine learning to enhance education.
Future trends in educational assessments
Future trends in educational assessments focus on innovative approaches that utilize machine learning and data analytics. As technology evolves, so does the way we evaluate students.
Personalized Assessments
One significant trend is the move toward personalized assessments. Instead of traditional tests, educators are creating assessments tailored to individual learning styles. This means students can demonstrate their knowledge in ways that suit them best.
Continuous Feedback
Another trend is the shift from one-time testing to continuous feedback. Using machine learning, educators can monitor student progress in real-time. This allows for dynamic adjustments to teaching methods and resources.
- Adaptive quizzes: Adjust difficulty based on student performance.
- Instant feedback: Provides help when students struggle.
- Ongoing assessments: Supports learning as an ongoing process.
Additionally, the integration of technology into assessments is set to rise. For example, using virtual and augmented reality can create immersive assessment experiences. Students can engage in simulations that test their understanding in practical contexts.
Data-Driven Insights
As data collection improves, schools will leverage big data to gain insights into student performance. This analysis will help educators identify trends and improve learning outcomes across the board. By understanding where students excel or struggle, teachers can refine their approaches.
- Predictive analytics: Helps forecast student success.
- Data visualization: Makes complex information easier to understand.
- Customized interventions: Targets students needing extra support.
Ultimately, these future trends in educational assessments emphasize a more holistic view of student learning. As we move forward, embracing technology will pave the way for more effective and engaging educational practices.
In summary, the integration of machine learning in education is reshaping how we assess student learning. Personalized assessments and continuous feedback create a more engaging experience. As we embrace future trends, technology helps us better understand and support each student’s journey. Overcoming challenges such as data privacy and integration is crucial for maximizing these advancements, ensuring that we provide the best learning environments possible for all students.
FAQ – Frequently Asked Questions about Machine Learning in Education
What are the benefits of using machine learning in educational assessments?
Machine learning provides personalized learning experiences, continuous feedback, and data-driven insights, enhancing student engagement and outcomes.
How can machine learning help identify at-risk students?
By analyzing data and performance trends, machine learning systems can detect students who are struggling, allowing educators to intervene early.
What challenges come with implementing machine learning in schools?
Challenges include data privacy concerns, integrating new technologies with existing systems, and ensuring teachers receive adequate training.
What future trends should we expect in educational assessments?
Future trends include personalized assessments, continuous feedback, and the use of technology like VR for immersive learning experiences.